AI Disruption: DeepSeek and Cerebras
Hello.
Bryan Cantrill:I think we got Andy and James.
James Wang:Hey, guys. Hello. Hello. Hey.
Bryan Cantrill:How how are you all? Thank you so much for joining us. This is, it's very exciting.
Andy Hock:Oh, you literally had to click invite to speak. We we sincerely appreciate the invitation.
Bryan Cantrill:Oh, yeah. No. No. Absolutely. Yes.
Adam Leventhal:Sorry it's not embossed or whatever.
Andy Hock:Yeah. That's right. You know,
Bryan Cantrill:you know, we we got, Adam, I'm I'm not gonna I'm not gonna spoil their surprise, but we've got a a mutual, friend who's getting married. And I was advising that person, kinda might have to, like, really I'm gonna have to hide this from you. I could think of it. I I don't think you've heard of this yet, about the wedding industrial complex and kind of the embossed invitations. Do you I I always tell, like, if you're getting married, it does not like, it does not matter what your invitations look like.
Bryan Cantrill:The the wedding industrial complex will try to get you care about this stuff.
Andy Hock:But Yeah. Please don't. I'm very concerned about the card stock the card stock that was used for our invitation. I think, you know, we can we there could there could be upgrades there. There's room there's room for it.
Bryan Cantrill:Yeah. But nobody cares. That's it. Nobody cares. Especially long after the fact.
Bryan Cantrill:So, Adam, I was I was talking to Andy about, about the possibilities of having, Andy and James on today. And, Andy had listened to a couple, you know, dabbled with a couple of episodes. He's like, I I kinda get it. I think it's like, Mystery Science Theater 3,000 for and I'm like, yes. That's exactly what
Bryan Cantrill:it is. But have we figured out if that is, like is that a reference that
Bryan Cantrill:is actually carried across the generational chasm? Have we figured this one out? I MST three k?
Adam Leventhal:I don't think so in part because I think they've I think it's, like, not showing anymore. But I I would say, Andy, first of all, that is, like, the highest praise we could possibly receive. We've even used MST three k as literally an image for for one of our episodes. So, like, you're spot on. The only thing that you could have been
Andy Hock:higher priced
Adam Leventhal:the the only thing that could have been higher priced is perhaps, like, our real goal, our real secret goal is car talk. Like, we are trying to be the car talk of technology.
Andy Hock:Oh, yeah.
Adam Leventhal:Like, the click and clack of tech. So we're we know we know that you got there, but, like, we're we're on we're on on the ascent. We're working on our our way up to to click and clack.
Andy Hock:No. I like that because it's also it there there is some technical content. Right? It's it's it's the it's the levity and the snarkiness and the conversational tone about it that I think was really it, but I was thinking about it actually just before this. It's it's almost like if MST three k had a journal club.
Bryan Cantrill:That's exactly what it is. That's exactly
Andy Hock:what it is. Thinking, you know, we're not making snarky remarks about something. It's it's just very conversational and also light and humorous. And so
Bryan Cantrill:That's that's exactly what it is. This is you know, these are. Thank you, Andy, for high praise. That is that's exactly what it is. You know us well.
Adam Leventhal:We'll invite you anytime.
Bryan Cantrill:Exactly. So we we we got here because, the and admittedly, there's been a lot several years have passed in the last week, but the, a a week ago, there was a lot of attention on this on DeepSeq and the release of their r one model. And, Adam, we had talked about DeepSeq, with Simon Wilson in our predictions episode. And the and had really I mean, I thought that the DeepSeek result released on Christmas was amazing. Yeah.
Bryan Cantrill:And just the fact that you had this kind of moment where, all of Silicon Valley was, stunned, furious, that that, someone, a much less resourced team had, pulled off something that was that was a better result. And, you know, Adam, it was funny because we had some college students by the office yester last week, and they were coming out because of their association with one of our investors, and they kinda tour a couple of startups. And, Steve, Steve Doc, our our boss was, he he made passing reference to CDC,
Andy Hock:and I'm like, you can't
Bryan Cantrill:no one's gonna know what control data is. You gotta, like, stop and explain that one. I mean, like, you know, Tomax and and and Zaimat and, you know, there's some of these other things may or may not have left the generational chasm, but I mean, come on. We this is, CDC has to be explained. So I went to get the the the letter that we have that our colleague, Josh Clulo, printed out when we first started oxide, Adam.
Bryan Cantrill:Adam. I don't know if you know the one I'm talking about.
Adam Leventhal:Yes. Yes. No. A %.
Bryan Cantrill:So this is a very famous memorandum, and I'm I'm gonna do a little out loud reading if you don't mind, because I think it's a good segue into deep seek. This is a a memorandum, from, TJ Watson Junior at IBM. So this is, last week, he says sending out this memo to his team. Last week, CDC had a press conference during which they officially announced their 6,600 system. I understand in the laboratory developing the system, there are only 34 people including the janitor.
Bryan Cantrill:Of these, 14 are engineers and four are programmers and only one person has a PhD, a relatively junior programmer. To the outsider, the laboratory appeared to be cost conscious, hardworking, and highly motivated. Contrasting this modest effort with our own vast development activities, I fail to understand why we have lost our industry leadership position by letting someone else offer the world's most powerful computer. At Jenny Lake, which is gonna be their off-site, I think top priority should be given to a discussion as to what we're doing wrong and how we should go about changing it immediately. That is 08/28/1963.
Bryan Cantrill:And, Adam, doesn't it feel like that could have been written by Mark Zuckerberg or Sam Altman, like, last week?
Adam Leventhal:A %. Totally. That's spot on.
Bryan Cantrill:It it is and it just felt like, oh my god. This is just what what is, what is old is current, and I mean, this is why, you know, you and I believe aren't believers in reading history because this stuff is this is not the the the first time certainly and absolutely will not be the last time that, we've seen massive disruption from from DeepSeek. So we really wanted to talk about DeepSeek and then I, you know, what's it like? Who's talking about it? How are they using this?
Bryan Cantrill:A bunch of interesting stuff out there on DeepSeq that I wanna get to. But, I found James, I think it might have been from you, but I found that Cerebras was using I'd had DeepSeq available on, had not only had it working on their machines, but had it available where you could actually go try it out. And I and this is and we'll we'll get into this about the the kind of the power of it being, an open an open weights model. And I'm like, this is great because I've I've been meaning to try this out. And, Adam, you tried it out.
Bryan Cantrill:Right? Did you try it out on on Cerebras as well?
Adam Leventhal:I did. So, I mean so we we should talk about what's I mean, I'm sure we'll get into what Cerebras is, but it is so ridiculously fast, especially for folks familiar with, like, ChatGPT or some of these other ones. Like, it's the fact that it just gave me the response instantaneously, make me felt like something was broken.
Bryan Cantrill:You heard it felt like?
Andy Hock:I love that reaction by the way. Thank you. Yeah. Well, I Good. Yeah.
Andy Hock:And and
Bryan Cantrill:well, this is, again, again, we can we can wonder about MST three k leaving the generational chasm or not. I think Out of This World has not let the did you ever watch Out of This World, Adam? I'm not. It's this terrible sitcom from the eighties with the alien who could freeze time.
Adam Leventhal:I do remember that.
Andy Hock:And so, she would she would you know,
Bryan Cantrill:I remember this. She's just like, it I've learned that it's easier if I say I remember this now. If I don't
Adam Leventhal:It gets to the end faster.
Bryan Cantrill:It just gets to the end faster. But she could freeze time and would, like, run around. And that's what I felt like with the with the with DeepSeek on Cerebras, I felt like you would ask it some question, and it would live a thousand lives in what seemingly instantly. Yeah. And, you would come back.
Bryan Cantrill:And so alright. So so I guess, James and and and Ed, I guess my my first question is maybe this is just totally routine for you at this point. But with the the the performance of it really does seem pretty mind bending. And, obviously, that's the whole point. But, were you surprised at the at the performance of DeepSeq on Cerebras?
James Wang:Not at all. This is James. Hey. Hey, guys. Not at all because it is a it's a 70 b llama model distilled that we that's the version we hosted on Cerebras Inference.
James Wang:Our claim to fame, basically, the last year has been running the fastest llama 3.3 model, which is the seven v version is probably the most popular developer model used across industry. Everyone will fork it, fine tune it. It's, it's, it basically gives you kind of like GPT four ish level performance on a set of weights that you personally can control. So our performance on that was just like around 2,000 tokens per second. And when we saw Deepsea came out, we're like, oh wow, This is amazing.
James Wang:But it's this very exotic six seventy one b MOE mixture of experts model. We can't just run that instantly. Oh, but wait. They released a distilled llama 70 b version. And that one is basically think of it as bitwise compatible with with what we host already.
James Wang:So we just swapped out the weights, did a little bit of optimization, and shipped it. And so the performance basically replicated kind of like our state of the art llama performance.
Bryan Cantrill:That is really cool. So I I mean and we talked about llama a year ago, but I assume I mean, this is not a deep thought, but the release of llama must have been, rather, earth changing for for cerebras.
James Wang:Yeah. Yeah. For sure. I would say before that we were, a very exotic computer company. If you wanna do your, old school, like, computer company analogies, like personally, before I joined Cerebras, I feel like I was joining the modern incarnation of Cray.
James Wang:Like, it was so exotic that people can barely comprehend how it worked. All the all the cooling and power was just completely bespoke and made for this exotic chip that was only one of a kind and to this day still is one of a kind. And that's all the upside. The downside is that it's extremely difficult to sell and very hard for customers to feel like they can buy one. Like the the distance of buying a Cerebras anything was the the the longest customer sales journey, quote, unquote, you could possibly imagine.
James Wang:But the second inference came out that that distance became as as short as buying an API call from Twilio. And that's that's how we basically kind of blew up in last year. Well, the
Bryan Cantrill:I mean, a very kind of visceral display because we said that, like, these open weights models are gonna be are gonna change many, many things. But I think one of the things that I didn't quite anticipate at the time, but of course makes subtle sense is, like, it's also gonna make it much easier for people with really disruptive hardware advantage to be able to express that advantage, because that you can get your own you can get it working on your own on on your chip. You, like, you don't have to leave it to the user to go do that. It Yeah. Is it worth it?
Andy Hock:It's it's it's exactly right. And it also I mean, I think one of the things that the community has has has observed about open models from mama to to DeepSeek and others is it also gives people an opportunity to to build on top of really, really readily. So, yes, I think, hardware companies can build up to them, right, get them get them loaded on easily and show advantage, but also gives developers the chance to to to do more with them. Right? And and more deeply understand what's going on underneath the hood.
Andy Hock:And so I think the open model ecosystem, I think, is is is gonna go nowhere but up, and become more and more capable. Yes. It definitely gives sort of novel hardware architectures like ours sort of a chance to to shine with state of the art capabilities. I also think one of the things that's interesting about DeepSeek, some some of the sort of specific speculation aside about resources, is that it it it also shows the value of hardware software codesign. Right?
Andy Hock:Yeah. Interesting. Building a model that acknowledges the back end on which it's built or on which it will run. And so I also think that, you you know, our architecture is a little different from a GPU. Right?
Andy Hock:We we built our chip in a very specific way to accelerate the the computational workload that is AI. That's not graphics. It's not ray tracing. It's not database management. And so ours, like, we we we saw the sort of hardware software codesign elements of what was done in DeepSeek, and very much appreciated it.
Andy Hock:And I think there's to that point, I think there's actually a whole lot more that developers, could do above and beyond the capabilities of DeepSeek by developing for a back end like ours.
Bryan Cantrill:Yeah. Interesting. Well, it or it's maybe it's just worth elaborate. You we've kinda hit around a little bit, but it is worth elaborating on what the the what you've done from a hardware perspective because it really is extraordinary. So you do you wanna elaborate a a little bit on wafer level design?
Andy Hock:Yeah. Sure. Happy to. So, you know, it like, this is the this is the moment in a conversation where were we on video, we would hold up the the wafer. Right?
Andy Hock:But, but the our the sort of the heart of our product and our technological innovation at Cerebras is the development of a different kind of computer chip. We call it the wafer scale engine. It is the biggest computer chip, ever made. It's more than 50 times bigger than the largest chip built before it. It is it's quite literally, about the size of a dinner plate.
Andy Hock:It's about eight and a half by eight and a half inches of unbroken silicon. Whereas your, you know, your typical computer chip is maybe the size of a postage stamp or, a fingernail. Right? So we are a massive chip, and then we're we're now in a third generation of producing chips and systems around that wafer scale engine. I mean, it's visually impressive, but we didn't build this big chip just because it looks cool.
Andy Hock:We built this big chip for a very particular reason and really from first principles by looking at the AI workload. So then you ask yourself, okay. Well, what is AI asking of the underlying compute? Quite honestly, whether it's training or inference. And the the short answer is AI wants a lot of flops.
Andy Hock:Right? It wants wants a lot of compute power, but not just any compute. Right? It doesn't really need 64 bit precision. Typically, we use 32 bit or 16 bit or even eight bit or lower.
Andy Hock:Right? So and and it's also not all dense compute. There's a lot of sparsity in, in AI compute. So generally, a lot of compute, but generally low precision and a higher degree of sparsity than normal, or than something like, say, graphics. Second, AI is really a problem of handling data in motion.
Andy Hock:Again, whether it's training or inference, you need those compute elements to be close to one another so that they can share data and move inputs and activations around. And you in addition to communication bandwidth, you also need a lot of memory bandwidth, And that's very, very important, particularly for generative AI inference, which is where what we're what we're sort of talking about today. But so basically, you need a lot of compute, but a particular kind, and you also need a really high communication bandwidth and really high memory bandwidth. These are the things that AI compute wants. And then you look at that chip, and it starts to make sense.
Andy Hock:Right? Compute, we've got 900,000 cores on that chip, a 25 petaflops of sparse FP 16 compute on each chip. Right? So the compute equivalent of a modest GPU cluster, but all on one chip. And the reason, again, back to the, you know, the big sexy wafer, the reason we kept it all on one chip is so that all those cores could be directly connected over silicon, not over network interconnect and, you know, centimeters to meters of fiber or copper cabling, but by microns over silicon.
Andy Hock:So, orders of magnitude more communication bandwidth, and also all of our memory is on that big wafer. So it's all on chip SRAM. Unlike a GPU, we are not reaching off to off board HBM memory every time we
Bryan Cantrill:Oh, it's not HBM. It's all SRAM.
Andy Hock:It's all SRAM. So literally orders of magnitude more memory bandwidth. And that's why we kept it all on one big chip. We actually made the wafer, as big as you possibly could. So we start with a 300 millimeter circular wafer, and we cut the biggest square you can possibly cut out of it.
Andy Hock:So that we can get as
Adam Leventhal:much I I do love
Bryan Cantrill:the fact it's, like, it is literally the largest way. It's, like, if they made 36 inch wafers, it'd be it'd be thirty eight six inches. Like, we're just it's, like, at this is the this is just the size that, this is what a silicon ingot comes in that that that size of a wafer.
James Wang:You wanna you wanna know something fun, Andy? I learned from Sean, our CTO, that it's it's actually not the biggest square you can cut out of the
Andy Hock:Oh, no.
James Wang:In the circle. It is actually slightly bigger than the circle.
Andy Hock:Oh, that's right. Because the corners aren't actually corners. The corners are
James Wang:a little bit round. Someone shared the eels blog that I wrote, like, two weeks ago.
Andy Hock:Yeah. Yeah.
James Wang:And you will see the side by side with the orange thing. Did I make the corners?
Andy Hock:You did make it. Oh, look at that.
James Wang:So so the square is actually larger than the the the circle. And I found this the hard way because because I was writing this blog, and I'm like, I did the square math of the surface area of a square, the the area of a square. You know? Yeah. And I did the circle.
James Wang:I'm like, hey, Sean. There's no way this square can fit inside the circle. Is our monthly numbers inflated? I thought, like, I discovered, like, some kind of, like, flaw. I mean, he's like, no.
James Wang:The square is bigger than the circle because you don't need the very tip of the edges, and we wanted every tiny transistor out of the whole thing. I was just like, wow. So that was my learning.
Bryan Cantrill:Yeah. That is amazing. And then so how much memory does the thing have?
Andy Hock:So we got, 44 gigabytes of all on chip SRAM in that All
Bryan Cantrill:on chip SRAM. Wow. That's
Andy Hock:Yeah. So what we do for for inference, for example, what we do is we'll load the full set of model weights into SRAM on one or more wafers, and then your model is just sitting there ready, waiting to answer your question.
James Wang:Yeah. Here here's why it's fast. I think people are like, okay. You have a big chip, but why is it really fast? The reason why it's fast is is because to the way these LLMs work, it's basically speed as a function of how many parameters you have.
James Wang:Right? If you have, let's say, in this case, 70,000,000,000 parameters to to generate one token or one word, if you will, you have to run through, every parameter in the model. So at some point, your ALU on your on whatever chip you have has to run through 70,000,000,000 units. Right? And you can't 70,000,000,000 in let's say eight bit integer is is 70,000,000,000 70 gigabytes of memory.
James Wang:And you can't store 70 gigabytes on any regular CPU or GPU. So what they do is they just copy the weights in batches over until it it it finally goes through all 70,000,000,000 parameters. But that copying, is done over your memory interface. But you would think that you just have to do that once, but the second you compute the next word. So when ChaiJiPT says, hi, how are you?
James Wang:And it's about to say you, it has to do the 70,000,000,000 all over again because it has to evict the old weights out to get the new ones in. So to this constant loading of the same weights, the exact same weights, the weights haven't changed, right? The weights are hugging face over and over again just to generate one word. That traffic is why it is so slow and why GBT comes out roughly a hundred tokens per second. For us, all the weights, which never change and you just you're just streaming them through us, sit entirely in SRAM right next to the ALU.
James Wang:And that memory bandwidth is a thousand times wider than the GPU memory bus. That's the fundamental reason why we're so fast, and there's no, like, neat trick that GPUs can do to to just catch up with us on this speed.
Andy Hock:This is one of the fun things actually is and and you can check this out and go on to say artificial analysis where they post data from different vendors, inference APIs and different hardware back ends and different models. And what you notice if you look at that data is that, all the GPU inference providers seem to sort of limit out at a at a certain level. Right? Like, even the best can only go so fast. And it's true because it's actually fundamentally bottlenecked.
Andy Hock:Generative AI inference performance is fundamentally bottlenecked on GPU architectures by that memory bandwidth link that James was talking about. And for us, we're just not bottlenecked by that. For for Cerebras, we actually compute bottleneck. Yeah. So it's a it's a fundamentally different bottleneck, which allows us to deliver a fundamentally different level of performance.
Andy Hock:So for a a model like, well, model like DeepSeq r one Distill LAMA 70 b, the one that we launched with here, right, we're 57 times faster than GPU instances. And that doesn't mean you could buckle together 57 x DGX h one hundreds and get that fast. You can't
James Wang:For any number.
Andy Hock:For any number of GPUs. So it's it's it's quite literally a GPU impossible level of performance that you can achieve with, with with Cerebras for these kinds of problems.
Adam Leventhal:That that's amazing. And you were saying that, you have 44 did I get that right? 44 gigabytes of SRAM.
Bryan Cantrill:Yes.
Adam Leventhal:And then but you need, so these models fit in the 44 gigabytes, or do you need to trim them in some clever ways to get them to fit?
James Wang:They so for a 70 gig model or 70 gigabyte sorry. Filming parameter model, it doesn't fit right because you need minimum 70 gigs. So we put it on multiple wafers. So we chain together multiple CS systems until we have enough memory to fit.
Bryan Cantrill:Got it.
James Wang:And and the the good thing is people are immediately like, oh, doesn't that mean your bandwidth limited? Because now you have to go off wafer. For inference, it doesn't because all you're doing, if you think of like these models are just like layers of a, of a lasagna or something. Right? The memory bandwidth you need between layers is actually very minimal.
James Wang:You're just propagating these, these activations across that doesn't need much memory. So the hardcore compute is really loading the weights over and over and over again. But so long as we store the weights on the SRAM, two of two systems, 10 systems, we can propagate it across like a pipeline.
Adam Leventhal:And this is an important distinction between GPUs where you're saying throwing more GPUs doesn't kinda help with some of these key limitations, whereas throwing more of your wafer scaled, chips would help with larger models, for example.
James Wang:Yeah. So the GPU unit of memory scaling is determined by NVLink, which is the kind of NVIDIA interface they designed to make GPUs connect faster, communicate directly with higher bandwidth. And the NVLink today basically maxes out at eight. The next generation product supposedly goes higher, maybe 72, but we haven't seen any benchmarks yet. The realized efficiency is also kind of a unknown until you measure it.
James Wang:I'm actually personally very interested to see how far they go, for the next for the for the new generation. But the current limit with GPUs is basically eight GPUs can be collected via mid link.
Bryan Cantrill:Yeah. Interesting. And then so and James, you described it when you kinda came to Sveribris, it felt like, okay, this feels like a supercomputer company. This gets a cray. Yeah.
Bryan Cantrill:Presumably because the the programming model for this, I would assume I mean, it's it's something it's not CUDA, I would assume. Right? I would assume you've got a a different kind of programming model to take advantage of the hardware. Is that is that right? Or the what what is the what is the software interface of this thing look like?
Andy Hock:Yeah. So so much to say here. So I think I think James and I probably both pipe in. But, but, yeah, I'm I I like to joke that I'm a recovering researcher. My my background is actually in in physics and algorithm development.
Andy Hock:So I I came at the world of computer system design from, you know, from from wanting to make, model training go faster so that people could innovate more quickly. But I think that what that meant for for me from a product standpoint is when I joined Cerebras, you know, we were we still hadn't really built the wafer. Right? A lot of it was, it was this was early. This was back in 2017.
Andy Hock:So we had, like, designs on whiteboard, various stages of prototypes, and we were really good at just getting started thinking about the software stack. And, like, one of the early principles that we adopted, I think it was the right one from product and engineering, was to meet users where they are. And so you're you're absolutely right. Right? Like, our our instruction set architecture is fundamentally different because our chip architecture is fundamentally different.
Andy Hock:We're not CUDA at the bottom. We have our own microcode instruction set, and we have our own compiler that sits on top of that. But what I mean when I say meet users where they are is we we have that instruction set in our own compiler, But then on we we integrate all the way up to ML frameworks like PyTorch.
Bryan Cantrill:PyTorch AI. Yeah.
Andy Hock:And so when you like, if you're a a GPU developer today, you and you wanna train on a machine, you just describe the model that you wanna train in standard PyTorch, and you use standard Python, scripting to describe, say, the training loop. Similarly, if you wanna deploy a model for inference on a machine, again, it's sort of standard software frameworks that you would be familiar with, for a GPU like PyTorch. What's really sort of interesting also about, inference is that many inference developers aren't even doing things like loading models anymore. They're just writing applications that hit an API back end where a model is is is ready waiting for their query. And so in some sense, I I think, you know, eight years ago when we started on this, I think a lot of people were really thinking of of CUDA as a moat.
Andy Hock:Right? Because back then, there were still people that were actually writing a lot of neural networks in CUDA. I think AlexNet was written in CUDA. But the the the the software developer ecosystem has evolved a lot since then. Like, nobody's writing in CUDA anymore.
Andy Hock:Yeah. There's still some lower level kernel optimization being done and fundamental r and d around mathematics and algorithms of learning that's being done at that level, which, by the way, you could also do on our machine. We have our own low level language. But the vast majority of our developer community today is using PyTorch and and hitting inference APIs. And and we are exactly there so that users can bring model descriptions from GPU land.
Andy Hock:They can run them on Cerebras. They can take the results that are output on Cerebras. And if they want to, they can go back and run them on GPU land. It was really, really important for me and for our entire team, I think, to make this box that otherwise looks very different and has an intentionally different architecture, make that as easy to use as a single desktop GPU. Just give them orders of magnitude more performance and power.
Bryan Cantrill:When that's always the challenge is like, where is the right abstraction? Right? And you wanna come in with an abstraction that is high enough that I can express my unique power underneath it, but is still like at the right level where somewhere low enough that you can actually bring your software on top of it.
Andy Hock:And I think that like, was it did did the I know the acquired guys, they, They
Bryan Cantrill:I mean, obviously, we love acquired. I think acquired did land Morris Chang, by the way, Adam. The, James and Andy, Adam, in in in our annual performance review, Adam gave me the stretch goal of landing Morris Chang on this podcast that I, I think I was beat to the beat to the punch
Andy Hock:by acquired. But I didn't I feel like they
Bryan Cantrill:talked about CUDA being a ten thousand year lead.
Andy Hock:Do do you mind if I Yeah.
Adam Leventhal:Yeah. In the NVIDIA episode. Yeah. I think this is Yeah.
Bryan Cantrill:Wait. I'm gonna make you my I'm not making that up. I think they said it's a ten thousand year lead, and you're like, what? Nobody has it.
Adam Leventhal:It's a long
Bryan Cantrill:time. Come on.
Andy Hock:2,000. It's a long time.
James Wang:Yeah. I wanna see the derivation for that. That's a the marketing guy I
Bryan Cantrill:find that Yeah.
James Wang:Interesting marketing claim, and I wanna know how you get to it. I I made a particular marketing
Bryan Cantrill:How do you get
Andy Hock:to it?
James Wang:I I, you know, when we announced wafer engines three, we did it the same day as NVIDIA GTC right across the street. And, my pitch was basically, if you wanna, if you wanna see what Jensen's gonna announce ten years from now, just come to the Cerebras event instead. The the if you map out the the log scale graph of the the GPU transistor count growth, and you draw a trend line in ten years, it will hit exactly the transistor account of a c s CS three. Right? So that's a quantified marketing claim, which which I think is, not too bad.
Adam Leventhal:Yeah. It's gonna be early off by a factor of a thousand, but Yeah.
Bryan Cantrill:It's it's still a good plan.
James Wang:But on the on the CUDA point, on the CUDA point.
Bryan Cantrill:Yeah. Yeah.
James Wang:All those layers, whether you're programming to to CUDA or, above it at at at at, like, CUDA libraries or PyTorch or llama, those are all valid levels of abstraction. Right? Any developer developing for an enterprise stack can develop as high and low as they want. The interesting question now is just which layer in that, in that stack is the most exciting and relevant? Where are, or the like, in other words, if you count all the developers, where do they choose where to go in that, in that elevator of, in that like stack of, of layers?
James Wang:And I think, you know, just following eternal truths of, of, the computer market, people go to the highest level of abstraction when developing the latest apps. The reason why we have more AI apps than ever today is not because everyone now has become proficient in CUDA or, or even has learned anything about CUDA. It's because the entire AI stack all those years, including the contribution that CUDA laid right at the beginning, I was there at NVIDIA when it was launched. I mean, it was very clear what was done. Right.
James Wang:But all that now has been abstracted down to a single API endpoint that you can call. Right? To develop AI in 2012 meant like MacGyvering your GPU drivers to think your shaders can do math for neural networks. That's 2012. Today, to be an AI developer, quote unquote, you call the OpenAI API key.
James Wang:You give it a prompt and it gives you a bunch of tokens, a bunch of words back. All of programming has all of AI programming has been reduced to basically Twilio text messages back and forth. That's the level of IQ you need now. AI developer. You don't even need to know PyTorch.
James Wang:Like most developers today, they're they're building, like, apps to do your to simplify dental records and then to cure loneliness. You don't need PyTorch for that. PyTorch is is something that someone has met
Andy Hock:at you.
Bryan Cantrill:Yeah. PyTorch does maybe cure loneliness in maybe a roundabout way, but, yeah. The okay. So the and but it must have been I mean, it even so, I mean, it it must when llama was put out there, again, that must must have been really changing your trajectory because you now have something that is unlike, you know, kind of prior to that, you would have to have encouraged someone to effectively train their own model. And now you've got the ability, but, no, no, actually, just use this thing for inference.
Bryan Cantrill:You can go download. I mean, was that was that as big a deal as it as it feels like on the outside?
Andy Hock:Yeah. So let me maybe I can give, like, a little backswing to that, and then James can probably carry it forward. But I think one of the things that we had been observing leading up to the release of my llama. First of all, of course, models kept getting larger, and I'm sure we'll keep coming back. We'll come back to that.
Andy Hock:But, you know, a a fun, for example, is between, I think it was 2018 and 2023 sort of in in the in the ML bounding box between something like BERT large and GPT four. The the compute requirement for models and and the models themselves, got about 10,000 x larger. So four orders of magnitude growth and model size over the course of about five years. So models were getting larger. And the other thing that was happening in the model domain is that the the community was was sort of reaching an equilibrium in architecture around these generative pre trained transformers, right, these autoregressive transformers.
Andy Hock:And so what that meant for us as a as a hardware company, particularly a hardware company that's investing a lot in software to make the system easy to program and use, is it meant that the the the underlying kernels weren't changing that much anymore. Whereas, you know, six, seven years before, people were still discovering attention is all you need. And so there there was a lot of sort of rapid evolution in the underlying architecture and compute kernels for AI models. And there was a stabilization in equilibrium like an equilibrium being reached in model architecture. And so that was already making things easier to your question because we didn't have to sort of keep up as quickly with our lower level kernel libraries.
Andy Hock:But then when when those model architectures were published, we could immediately implement them and Llama getting the sort of attention and excitement in the developer community around it just gave us a really, really obvious target to to to to bring up, and and use to show the world the power of the right processor architecture for this work.
James Wang:Yeah. I'll I'll give my outsider view of an outsider person inside of Cerebras' view, which is that I think I think with Lama inference Cerebras basically found product market fit. And before that it was, it was very, very hard to convince someone to, to buy a box. Right. That's just, with llama, basically we could compete the unit, the, the unit of demand became completely standardized, which is, I wanna buy a million llama output tokens.
James Wang:And and for the first time, we could just sell that thing to you. Right? We could sell the thing that you wanted to buy on your basis to you with a special performance differentiation, which is we'll run it 20 times faster. How about that? And that become a very, very compelling proposition.
James Wang:I think Llama, I think just the the the call it the dominant model tokens as the product has basically kind of like standardized AI in terms of what is the unit of sale and what is the unit of consumption. That has been a complete godsend for computer providers Cerebras and, and all our competitors. Right? Because all of a sudden we can just compete on that basis. We didn't have to give you a custom pitch about our stuff.
James Wang:You could not care about wafers or anything and still find our product compelling. Because in the end, we deliver you a bag of tokens at a speed you've never experienced before at a price that's very compelling. So that was like Yeah. And just the
Bryan Cantrill:ability the the ability to get way up the stack of abstraction where it's like, you know, yeah. No. We're not we're not even talking about PyTorch anymore. We're talking about the thing that you actually want, which is the ability to actually
James Wang:Is that to
Bryan Cantrill:execute these models.
James Wang:No one who buys a bag of llama tokens ever asks you if you support CUDA. It's just not never a question.
Bryan Cantrill:Right. Right. When you and, honestly, like, you don't know when you when you go to these back ends, you actually don't know what you're actually and you can folks are running them on CPUs now. I mean, it is actually it is remarkable and just these getting these open weights models out there allows people to into implement them for a bunch of different platforms, which is really, really extraordinary. Okay.
Bryan Cantrill:So that was in the llama world, and then, DeepSeek kind of comes out of nowhere. I think even I mean, that's my read on this. It's I mean, I I it's, you know, Simon Wilson, for him, I think it came out of relatively speaking out of nowhere, and I think Simon's got a pretty close eye on these things.
Adam Leventhal:I don't think even relatively out of nowhere, Brian. I think our I remember the it Out of literal nowhere? It dropped well, it dropped on you on on GitHub with no documentation. It just sort of Right. I mean, it was just a a message in a bottle kind of thing.
Adam Leventhal:And there there was documentation that came the next day. And I think it was on Christmas day or late Christmas Eve or something. But yeah. Like, I think very much out of nowhere.
Bryan Cantrill:Yeah, you know what it, it reminds me of, an ouroboros. I don't know if I'm pronouncing I'm sure I'm pronouncing that incorrectly given the number of things I pronounce.
Adam Leventhal:I think you're pronouncing it correctly.
Bryan Cantrill:Do you know what I'm talking about?
Adam Leventhal:Yes. A snake eating its own tail?
Bryan Cantrill:A snake eating its own tail. And do you know the well, I'm not sure if it's or if if it's pronounced correct right now, but do you know where I'm going with this? This is the the the language Ouroboros that there was a so when we were doing development of LX branded zones, when we wanted, like I wanted to get as many Linux apps as possible. I mean, it's a bit of a serious problem. Just like, okay, I'm trying to run a a I'm trying to provide fidelity with kind of software that's out there.
Bryan Cantrill:And there was just your your point about this thing just like appearing on GitHub without any comments. There there was this ouroboros that had that was a hundred different languages in a ring, which is to say that the first program in the ring would generate the second program in a different language, which would generate the
Andy Hock:Do you remember you called
Adam Leventhal:this a quine? Is that is that the same
Bryan Cantrill:It's a quine. Yes. It is a quine. Yes. That's right.
Bryan Cantrill:And the the the the the the Ouroboros is a 100 language client that's gonna ring. K. And the but this thing appears on GitHub with no comments, and people are like, how did you do this? Who are you? And it's like, it was just someone in Japan who's just like and someone people ask me, no.
Bryan Cantrill:Like, seriously, like, I'm a researcher. This is kinda like an open question. Like, how'd you do this? And they'd reply like, oh, you know, the cherry blossoms are lovely. It's like, okay.
Bryan Cantrill:Just like this. It's the Internet, man. This is what happens on the Internet. Like, sometimes you get people coming. So and I think that that DeepSeek kinda came out of nowhere in that regard.
Bryan Cantrill:Right? It it, like the coin relay, the the Ouroboros hundred language coin relay kind of drops out of nowhere. It sometime late last year, I mean, I I saw it when they dropped their I I think the Christmas model that they dropped was I know. Maybe James James or Andy, maybe you know when they that was the first time I'd seen it was when they dropped the model on Christmas day.
James Wang:They had a prior model called v three before. That was also very impressive.
Bryan Cantrill:Okay.
James Wang:That's the base model. That came maybe a few weeks earlier, and people were quite impressed. But but not I I'm honestly a little bit surprised by the level of reception with r one because even like, what what does it do? It replicates OpenAI's old series of models. Right?
James Wang:When old models dropped, it didn't create this much buzz. So I'm surprised that, like, the derivative created this much buzz. But, yeah, they, dropped around Christmas. I thought it was neat. I I watched it, but the the the the way it blew up over the next couple of weeks is pretty wild.
Bryan Cantrill:Well and I think so I think there well, what's your take on why it created buzz? I think there are a couple of reasons from my perspective that it created buzz, but why did this create buzz where o one maybe didn't?
James Wang:I think that $6,000,000 training cost was actually a huge part of the story of what made it, like, buzzworthy. That made it not just a technology story, but a financial story. And when NVIDIA nuked, like, 20% the next day, it became a global story. Like, it
Bryan Cantrill:It became a financial crisis.
James Wang:Yeah. Basically, financial crisis. I mean, the funniest tweet I saw was, like, there were two two lab leaks out of China, COVID and r one. And I'm like, yep. Those are the highest economic events in recent memory or for worse.
Andy Hock:Yeah. So I think maybe there's there's a couple of things. And there's there's a lot of good discussions about this, elsewhere. So I won't try to replicate those. But I think one is the question of training costs.
Andy Hock:And I do think there's some there's some genuine questions about that. And that's that's maybe a longer discussion in and of itself. Not specifically about deep deep deep seep, but how we as a community talk about the dollar cost of training. Right? I think one thing that that that commonly comes up here is, you know, we we often talk about this sort of the the one time production run.
Andy Hock:Like, how long does it take to train your model to convergence once? The fact is that that's that's not even close to the the total cost of training. Most production models get built, including this one. Right? Have there was there was hundreds, maybe even thousands of, predecessor experimental runs that that that would lead to a model like this.
Andy Hock:And so, you know, I'm I'm not sure exactly what the the training cost is here, but but certainly, it seems to have been significantly less than prior models. So I think that was a big part of it. The other part of it, I think, that is noteworthy is the architecture itself. Right? It's moving towards this Yeah.
Andy Hock:Mixture of experts model, which, in in and of itself is a form of sparsity, or it's sort of expert sparse model, which means that it's, it's it's more computationally efficient, for a given input output pair. And I think, you know, I I was just in, we you know, there's a there was some some discussion on the chat earlier about, you know, how, weeks seem like years in terms of what happens in AI these days. The the the week before we launched DeepSeek, I happen to be, as it were these days in Davos for the World Economic Forum where AI was a big point of conversation the whole week. And a lot of it oriented around compute and how, you know, these how state of the art models require large scale infrastructure. By the way, I still think that's entirely true.
Andy Hock:It's still true in a deep in a post deep seek world. But it's also the the conversation wasn't just about amount of infrastructure. It was also around how computing infrastructure for AI needs to be more efficient, more sustainable, more accessible to a global community of of developers. And I I wanted to add to that each time the conversation came up that, yes, the computers need to be more efficient and but side note, ours is, but also the learning algorithms need to be
Bryan Cantrill:more efficient. Right? Yeah. Yeah.
Andy Hock:We use a lot of the development that that I referenced earlier between, say, BERT and GPT four effectively is is like a brute force approach to models getting smarter. But I I I think as we go forward, one of the things that I found striking and and sort of prescient, hopefully, about deep seek is that models are getting more capable while not growing linearly in size or compute cost. That is, we're figuring out how to build models that are more efficient learners and more efficient execute of their work. And if we do that as a community that has built smarter models and learning algorithms and we build more efficient computer systems, then I think we start to wrap our arms around the sort of the the energy cost of AI conversation.
James Wang:Yeah. So just to add to what Andy said, like, if if we didn't have this, so o one and r one, these these new reasoning models, the the key thing they do is instead of spitting out the most likely token to answer your question, it spends some time gestating and thinking and then gives you the answer afterward.
Andy Hock:Right? That's the Right. Thing.
James Wang:And the Yeah.
Bryan Cantrill:In the word of by cloud, it's got a great explainer on this where he calls it yapping, which I I like. They they they they yap a bunch.
James Wang:Yeah. Exactly. They have a bunch. The key thing is you could get model intelligence by doing pretraining with larger and larger model sizes, or you could just, with a model size, think longer. And these two are interchangeable.
James Wang:And that's the key, like, new thing that's been established for, for large transformers. And the benefit, one way to think about why that's valuable is to say, okay, let's say we didn't have this, test on computer or reasoning, kind of style. What kind of a traditional model at what size would you need to match DeepSeek r one performance? Like, let's say g p four is 1,000,000,000,000 parameters. How many trillion parameters do you need to match r one style performance?
James Wang:And the answer is likely, like, maybe no amount will be sufficient. Maybe there's a very large number that would be enough, but it might be a hundred trillion parameters, a thousand Yeah.
Bryan Cantrill:Right now. Right. Doesn't make sense.
James Wang:Maybe yeah. There may be no size that's practically large enough to get you this performance, which would mean you you would need the kind of Sam Altman how many trillion dollars of investment to build a new fab kind of situation.
Andy Hock:That would be the old world.
James Wang:Right? With the new world, it turns out it's way more efficient just to think longer than to pre bake more weights into your original model. So you shift basically the compute burden from training time to inference time, and it becomes a lot more efficient.
Bryan Cantrill:Yeah. Well, and I think to a lot of technologists, like the idea that we were maximally efficient with these larger and and larger training rigs and larger and larger models, it's like there's there's a ton of efficiency. I mean, we just it felt like the focus was so on on kind of frenetically building these larger clusters rather than really looking at how they're used efficiently. I think a lot of technologies like, wait a minute, time out. Like, this this cannot be someone is gonna come along and is gonna do this more efficiently.
Bryan Cantrill:I love I just dropped a link to to a tweet that Eric Meyer had. Did you know Eric Adams? Eric is Eric is delightful. At some point, I'm gonna Eric has told me that he doesn't do podcast as a point of principle.
Andy Hock:He doesn't he doesn't listen to them, so
Bryan Cantrill:he refuses to be on them. So I'm not worried about him hearing what I'm saying about him now. Eric is very
Adam Leventhal:We just tell him it's a Discord call, and we don't need to tell him it's recorded.
Bryan Cantrill:Try talking about Discord. At some point, I'm gonna get Eric on here. I'm gonna I'm gonna figure out how to fucking get on here.
Andy Hock:Guys. Maybe send him, like, a an invite on some really nice card stock.
Bryan Cantrill:Oh, there it is. There you go. A little callback.
Andy Hock:Wedding industrial complex.
Bryan Cantrill:Tomorrow. Yeah. Exactly. The wedding industrial complex advice. We okay.
Bryan Cantrill:Yeah. That's fine. I'm gonna you're right. I'm gonna, I'm gonna invite Eric to a wedding that is actually a podcast episode. Eric is delightful delightful technologist, but he and he's done a lot of interesting things.
Bryan Cantrill:He did link dry at at Microsoft, back in the day, Adam. And but Eric has got this the it talks about at Meta being kind of just insulted by how inefficient these things were. And he's like, I'm gonna actually gonna do this much more efficiently. So, I'm actually like this I know this can be done much more efficiently, gets together a team of a team of renegades to make this much more efficient is showing a lot of, like, really great forward progress. And then, this is just what I love.
Bryan Cantrill:The so he's he's gonna be able to train these things much more efficiently with this much smaller group of people. And then, meta kills the team as part of its year of efficiency.
Adam Leventhal:Which is like
Andy Hock:Totally learned.
Bryan Cantrill:It even theme. Silicon Valley 1 act play. It's like we have, and which I I mean, it's just like it's this like classic innovator's dilemma. Right? And the classic kind of it's like you're gonna no.
Bryan Cantrill:No. You're if you succeed, you're gonna show that we've spent far too much money building this other thing. So we're just gonna,
Andy Hock:I I I, I'm hesitating to make a prediction, but I, like, I imagine we look back on on this on this decade of AI development and say that this was sort of the proof of concept phase and in some sense, the proof of value phase. Yeah. You actually needed to get, like, to to GPT four and to chat GPT, I think for for the world to take notice. When ChatGPT came out, right, AI became a topic of conversation around people's dinner tables. I think that was important for social discourse and for sort of general market awareness.
Andy Hock:But moreover, sort of enterprise fortune thousand CXOs had that sort of light bulb moment instead of, oh, you know, I even though I'm not doing search or ads, or or streaming, there there's a or a recommendation. AI I I could use AI in my business to drive revenue or efficiency. And so it it I think there's there's some value to that brute force, but to your point, there's so much overhead. Right? Like, we we raced to a point where we could show value and show that the concept was was valid.
Andy Hock:I think we've reached that point now. And so there's a very natural next step, which is to say, how can we be more efficient? I think there's tons of overhead there. Well and I
Bryan Cantrill:think, you know, we see this again and again and again in technology. It reminds you of supersonic flight. Right? When, you know, when when we were kids, supersonic flight was gonna be the way we we went everywhere. It was kind of like but as it turns out, it's like, actually, it's there are a whole bunch of reasons why that doesn't make economic sense.
Bryan Cantrill:And we're actually wanting but that which is not to say that aircraft development stopped. It just it moved in a different and much more important direction in terms of making it much more efficient to operate.
Andy Hock:Yeah. And now we're in the we're we're sort of in the infrastructure development phase, right, where we've we've proven the feasibility, we've proven the value, or in the process of proving the value, depending on how you think about it. And now we're in the process of building up the tooling, such that this capability can be integrated with more applications, business processes, our daily lives, etcetera. I found myself, thinking the other day in the shower because, you know, this is what we do. The other day in the shower Andy.
Andy Hock:But Yep. James is, like, making the the stop now motion. Right?
Bryan Cantrill:We we actually on our podcast episode, like, not even too recently, we talked about double headed showers on here. So this is like, you haven't gotten close to weird from our from our perspective. Okay.
Andy Hock:So I I only had one head of the shower on at this moment just to clarify it.
Bryan Cantrill:Okay. But but but careful.
Andy Hock:But yeah. Hold on. I was thinking to Shadi the other day, like, how do we as a as a community and as a society avoid AI becoming the flying car of, that that was sort of the the future of transportation envisioned by the, you know, the nineteen sixties and seventies and eighties. Right? It's not that flying cars weren't technologically feasible.
Andy Hock:They they they, I think, you know, there's some way that they would work and, you know, local air transportation may yet become a thing. But a lot of it was because of the cost and the infrastructure just didn't exist. Right? And so I think we're in that phase now where the we've proven the the feasibility. We've proven we're in the process of proving the value.
Andy Hock:And now what we're seeing is is the world rallying around billing of the infrastructure to make these things more, make these capabilities more accessible, more customizable, easier to integrate with your business applications. And I think back to to the topic of the podcast, right, that's also where, open source is is a huge thing, right, because it it makes these, capabilities so much more accessible to a broader world of developers, and that's laying down the infrastructure that will allow, you know, this proverbial car to really take off.
Bryan Cantrill:No. I think you're exactly right. They I mean, we the the economics kinda have to change have to have changed because and if you just built this with these large clusters, it's like none of these these technological revolutions have all of the advantages of the revolution accrued to the provider. That doesn't make sense. Like, it doesn't you can't and I mean, the advantage has to accrue to the user of the technology.
Bryan Cantrill:That's that's the only way it gets liftoff. And it has to be like, no, this is, yes, like, I'm, you know, maybe, I mean, I can, you know, provide for a Cerebras certainly, but I I it's like Cerebras can't take all of the advantage of LOMs. The advantage of LOMs ultimately has to live with the person who's using it. And I think in order to get there, like, we had to kinda hit these the I mean, just like with the I mean, if we had not had open source, we we a proprietary software world was gonna hit a ceiling, kinda had hit a ceiling, and you couldn't build a lot of the things that we have built in the last twenty years were not for open source. We needed open source in order to be able to do that.
Andy Hock:Yeah. I think those you know, the proprietary models are obviously extremely capable and in in in some cases far better at particular tasks that they're designed for. But to your point, that's that's a sort of one to one to one or one to end kind of, customer provider relationship. You you have to come to me if I'm a provider of a proprietary model, and we have to work together under very specific terms. Whereas, if if I'm an open source model, it's a sort of a one to many model, where, you can you can run it anywhere.
Andy Hock:You can tune it for your own purpose, and it becomes much more accessible.
Bryan Cantrill:Okay. So, Annie, let me ask you this. Because, obviously, the fact that it's an open weight model, there are two I mean, there are many enormous advantages. One of them is, of course, I can run it on my own infrastructure that I don't have to feed all all of my data to to this kind of third party, and I can I can keep that all on premises? And then there are these other advantages around my ability to innovate around it.
Andy Hock:Do you which of those
Bryan Cantrill:do you think is the do you think the ability to innovate is ultimately the more profound or I mean, how real is this desire to be able to to, not actually have to give my data somewhere else for fear of it being trained upon or what have you?
Andy Hock:I think I mean, it's it's hard to tell which one of those is gonna be end up being the the more impactful for the market. I genuinely believe both are. I think there is a very real concern of enterprise customers that we talk to about data proprietary, data privacy, data sovereignty. Right? I I think, it was true ten years ago, and it's even more true and more obvious to enterprise leaders today that, data is one of their crown jewels.
Andy Hock:Right? Whether whether you're an ad company like Google or a a health care company, like the Mayo Clinic, you realize now every single day that, your data is your value. And so I do think that, enterprise leaders and developers are becoming much more cognizant of the value of data for their work, and therefore, they're becoming more and more sensitive about where it goes and and and and what it can be used for. And I think that's that sort of, development trajectory is is only going to continue. On on the flip side, so I think that's that is a real concern.
Andy Hock:Right? And and to be quite candid, I think And
Bryan Cantrill:I would say you also have, by the way, a concomitant breakdown in trust where I think that people don't I I don't trust third parties to not train on my data. I just think that my data is too it's just too tempting. It's just sitting there. It's like, you know, it's like leaving food out with teenagers, speaking as a parent of several. It's just like, you just cannot expect the food to be out there again when you come back.
Andy Hock:Yeah. That's exactly right. And and to be quite honest, in the in in the earlier phases of our business, in fact, training is still big, big, big, big chunk of our business, whether it's delivering boxes, the data centers, or, customers training models on our cloud. Training is still a big part of our business, and a lot of customers come to us because they want to be able to build state of the art models with their own data. They and and they wanna be able to do that in a secure environment with a company that's not gonna go and and and use that data for some other purpose.
Andy Hock:Right? We're not we're not trying to build competitive models to our customers. And so customers know that they can come to us and they can get best in class infrastructure. They can get a model development partner, and they can roll their own or back to your point about open source, they can fine tune their own
Bryan Cantrill:model. Right. Right. Which feels like a much more viable trajectory for for many folks for that kind of long term.
Andy Hock:It is. And now they have that optionality. Right? They can they can, pretrain their own if they have that data and and if there's a clear benefit. Right?
Andy Hock:Maybe they wanna pretrain a relatively small model for a very, very specific purpose that doesn't involve, human language web web text reasoning. Right? There there's definitely a world where, where people may wanna build models like that, and they do. But to your point, maybe I wanna build a model that already has a general purpose understanding of, of language and reasoning, and I wanna fine tune it and maybe distill it to do something else, or do something very, very well. Right?
Andy Hock:Go to grad school in the topic, after it's already got a baseline education. And so I think back to your question, right, the the other massive benefit of of open source is this this developer accessibility and, the ability to build things really quickly.
James Wang:One easy way Sorry.
Bryan Cantrill:Just like the idea of sending an AI to grad school and, like, what do you mean you, like, you became a nihilist and then you spent, like, eight years on your dissertation and you've got, like, you're just holding odd jobs. Like, what are you doing? Like, you told me to go to grad school. I don't know. I mean, I'm like, with the the
Andy Hock:That's right. That's right. The the model just want we we wanted to give the model some time to avoid real life and go on too many Right. Right. And and and and and go into debt also.
Bryan Cantrill:Right. Exactly. Like, now, like, the model is, like, bitter about, like, you sent me to grad school. You told me this is gonna be a good idea. Now there's, like, do you realize, like, there are no faculty positions available anywhere, by the way?
Bryan Cantrill:And, like, thank you for having me study.
Andy Hock:The the
Bryan Cantrill:I'm not
James Wang:sure if
Andy Hock:you show
James Wang:up to class the first day and say, I've seen all these tokens before. What am I doing
Bryan Cantrill:here? Right.
Andy Hock:So the another
Bryan Cantrill:thing I wanna actually, what, Devine Rao had this take today, which I thought was interesting. I just just dropped in the chat, predicting that that the closed AI model providers are like, they they're not gonna an API can't be their endpoint, and that the that you're only gonna have open models via APIs. And it was because I was looking at this, and Navin Rao, you two should know, is is was my suggestion for being the next CEO of Intel. So we we I think it's safe to say that that's not gonna happen. The but, James, I saw that you had a reply to this, so I know you saw it.
Bryan Cantrill:What was your take on on Naveen's take there?
James Wang:I thought Naveen's take was interesting. I wasn't sure what to think of it. That's why I was, like, we're chatting back and forth. His view is that the model has kind of reached the point of commoditization now where all the capabilities are kind of on par. There is no model that's 10 times better than any models' model.
James Wang:Even the open source model is as good as the closed one. So there's no edge to doing that anymore. And so the value will move to the app layer. And that's a pretty popular EC thesis right now. Yeah.
James Wang:It is. Yeah. I I'm just having a hard time visualizing what that means. Like, is OpenAI gonna train this model, use it on their own, but not have an API so you just buy the OpenAI Samantha or super high level software thing? And if so, doesn't that imply you still need a powerful foundation model underneath?
James Wang:And that still needs to have an edge for you to sell a, like, consumer product to have an edge. Right? So if there's no edge in foundation models, wouldn't you just use the the best open source model like you you'd use the best open source Linux distro to build your company since there's no value there. So I haven't quite settled on what that means.
Andy Hock:I think so.
Bryan Cantrill:Maybe I was reading too much into this, but I felt what he was kinda saying is that the closed models are kinda dead walking. That that the models are that in order for models to be I mean, honestly, just like the the open source software didn't make proprietary software go away, but it definitely, changed the scope of prior proprietary software pretty draft drastically.
Andy Hock:Yeah. You know, I hadn't, I I I like where you're going with that and the the dead walking is a a far better metaphor than I was gonna give. So, but I like, yeah. We we we know Naveen, love what they did with Mosaic, and, and and I like his perspective here, actually. I think, do
Bryan Cantrill:you think about him as the CEO of Intel?
Andy Hock:I mean, I I want we
Adam Leventhal:get more channels. Why do you want that?
James Wang:Why do you want to sentence him to that?
Adam Leventhal:Oh, just a bit for the pride of being right. I mean, that's that's
Bryan Cantrill:Yeah. Exactly. No. No. No.
Bryan Cantrill:This is fair. This is fair. It's like, if we
Andy Hock:have to get to the meeting, why would you, like yeah. The meeting
Bryan Cantrill:is like a regional person. You're right. You're right. Of course. No no one deserves that date, which part of the reason why my prediction was that no one gets to that date.
Adam Leventhal:Anyway No one no one has signed up for that fate.
Bryan Cantrill:No one has signed up for that pay.
Andy Hock:I I think you do a backup job. And, and and and I do I I genuinely want for for Intel to be an incredible American success story. Right? Every every reason they could be. It does need the right leadership.
Andy Hock:Okay. Yeah. But, back to this whole the whole open source model thing versus closed source. I I actually still believe that there's gonna be a huge amount of value for for closed source models, but I think what the what what both what, like, models like, Wama four zero five, even before DeepSeek showed and what DeepSeek showed again, is that it it's not the only advantage. That is access to proprietary data and massive training infrastructure.
Andy Hock:Building being that is being able to build an accurate model isn't the only advantage anymore. You can't lean on just that. Right? NVIDIA can't lean on just CUDA anymore. The world has evolved past it.
Andy Hock:And I so I do think there's gonna continue to be, lots of good reasons for closed source models. I think they're gonna continue to be really performance and accurate. But, the the open source is gonna be just as accurate, just as capable. And so I think the challenge for the the closed source model builders is going to be how to deliver value beyond the accuracy of the model. So it's gonna be about Right.
Andy Hock:Products packaging. It's gonna be about go to market. It's gonna be about your relationship with your customers, your delivery mechanism. It's gonna be about that. Right?
Bryan Cantrill:And they're gonna have to be more efficient. Right? And they're gonna have to they can't ignore the efficiency gains of this. You can't kinda pretend that it's not happening. You're gonna have to don't
Andy Hock:you think that there's gonna have to
Bryan Cantrill:be much more focus on efficiency?
Andy Hock:Yeah. Absolutely. Absolutely. In fact, you know so coming back to the the, like, a closed source model that I think is is actually viable and will work in the long run. We have a a public partnership, actually with both, GSK, GlaxoSmithKline, and the Mayo Clinic.
Andy Hock:Right? They they're building models that are that are bespoke for a very particular purpose, whether it's drug development or things like hospital administration. Right? Like, they're building a model for a very, very particular purpose. And it doesn't necessarily make sense to to start with, some existing open ways model.
Andy Hock:But in other cases, it might not. Like, if I'm building a model for electronic healthcare records, I'm not sure if it needs to start from an open source web text English model. Right. This might not be even the best example, but I'm sure you get the point. Nonetheless, that that, you know, that's gonna end up being a a a model for a very particular purpose.
Andy Hock:And, again, it's not the open AI business model, but, like, I think we're gonna have a lot of enterprises, that will end up building proprietary models for their own purpose.
Bryan Cantrill:The right. And so less general purpose in in other words, much more special purpose. I mean, the Mayo Clinic question is a really interesting one where it's just like, no. This is not I I this is not something that OpenAI is gonna do. This is something that I I've got all the data.
Bryan Cantrill:I know how to I'd like I wanna train on it. It does not make I don't doesn't make sense for me to make us open weights for, I mean, many, many reasons, obviously.
Andy Hock:That's right. And that's like nobody knows you know, we talked to enterprise customers for a long time. Nobody knows the idiosyncratic business challenges or business problems that they have than they do as operators.
Bryan Cantrill:Yeah. Right.
Andy Hock:So I think they they are in fact and nobody necessarily has the data that they have, particularly in in the world of enterprise. So it it actually positions them really well to build, you know, the right model to solve their specific problem.
Bryan Cantrill:So the the other thing I wanted to ask you about about with r one, because it it's it's the the chain of thought, it offers up its entire chain of thought, which the OpenAI models don't. The OpenAI models are much more circumspect. And, Adam, I mean, I know you and I had to say I mean, we had similar reactions anyway. It is wild to look inside of what r one is thinking.
Adam Leventhal:But especially because with Cerebras, it vomits it out all in one burst. So you're kind of getting you're you're getting the showing of the work, which I was describing to Brian earlier. Like if I were in a conference room and I found these notes lying around of someone's inner monologue, I would, like, leave the building. Like, it is borderline like a pathy. And and I love the parts where it feels like, oh, like, shoot.
Adam Leventhal:I gotta say something. It's been a while since you asked me that question. Like, say something now, like, kind of panicking in this
Bryan Cantrill:It it does seem to panic. And it, like, it second guesses itself. It panics. It also, like, have you caught it, like, you know, it was hallucinating somewhat rampantly for me on, you know, it was doing the the, you know, the kinds of things that they do where it's, like, they're hallucinating the kind of APIs that really should exist, but don't. So you're spending your time being like, I knew that ex okay.
Bryan Cantrill:That does exist. And you're like, no, this doesn't exist. Like, where is this thing? And then you are correcting it. And then in its chain of thought, it's like, oh my God.
Bryan Cantrill:He knows that I actually don't know any of this stuff. I just like made all this stuff up. Like, I better go. I mean, it's just like
Adam Leventhal:I I asked it to write a paragraph about this show tonight, and it's like, DeepSeek? What the heck is DeepSeek? I don't know what it is. It might I say him right now? Oh, okay.
Adam Leventhal:I think it's something that Cerebras developed. Yeah. I'm gonna go with
Andy Hock:that. It's good.
James Wang:Jeff, it's a lot.
Andy Hock:Right? No. Yeah. It
Bryan Cantrill:I mean, James, Andy, was it kind of because I I don't think that there are other, I mean, it it it feels like it's on the leading edge anyway of OpenWeights, chain of thought models. Certainly, it's giving you more than you get out of OpenAI. I don't know if it would did you go did you all have the same kinda reaction to it? It is it's definitely wild.
James Wang:OpenAI has their model will do the same thing. It's just that they hide it. Right? Because they don't wanna hide it. Right.
James Wang:Exactly. Each release, they have be more protective of their secret sauce because they keep getting open sourced. So with the o one model, they're they're like it barely told any the world about what they were doing only to say that they use test time compute. Right. And then and then they hide the intermediate steps so you can't copy the chain of thought patterns.
James Wang:Because the thinking was if they showed you the whole thing, you could just scrape that data and use it to to train a fine tune model that would do the same pattern of thought. Interesting. So they did it, and and they thought, okay. At least, you know, they're pretty much we're somehow protecting this. But but DeepSeek, they they they figured out a mechanistic way using reinforcement learning to do it anyway.
James Wang:That's kind of early answering your earlier question. What was so impressive about it? No one thought you could just do pure RL and stay coherent, somewhat coherent in this chain of thought.
Bryan Cantrill:And That's what they did. Somewhat coherent. It is definitely somewhat on the rails. And then of course, on Cerebras, it's very fast. So it's it comes across as just manic.
Bryan Cantrill:I don't know. It's That's right. I'm with you. It's just It's manic in a way that
Adam Leventhal:OpenAI is not. It's just like The kinda open API teletype of it is more reassuring. It's like a person kind of cogitating through something as opposed to the instantaneous, self doubt is is, more alarming.
James Wang:Yeah. Well, yeah.
Bryan Cantrill:I just like I feel like it's like the scene from Breaking Bad or whatever. This guy just like this model just, like, snorted a bunch of meth is now screaming at me. But it's actually, like, actually, it's got some good ideas, though. It's got some it's, you know, got all wrong. It's got some interesting stuff.
James Wang:In big five speak, we call this high neuroticism. Yeah. Mhmm.
Andy Hock:That's right. And and not necessarily in the direction of of of more neuroticism or, or meth or self doubt. I think one of the things that I find interesting about this and illustrative is that as you start to play around with these very, very capable reasoning models, you start to realize that like doing it on old infrastructure is not gonna work anymore. Somebody in the chat earlier made some reference to like dial up versus, versus broadband. And Yeah.
Andy Hock:One of the things that one of our, one of our PMs here said a while back is that generative AI is in the is in the dial up era of its evolution.
Bryan Cantrill:And I think Yeah. I've noticed that
Andy Hock:is because of of the the the underlying performance of of hardware. Right? Like, people started playing around with Gen AI models and I'm like, oh, these are pretty cool. But then as soon as you wanna try to put some large reasoning model or some multi agentic workflow or even a a big capable multimodal foundation model and and run it in inference time on a GPU, you're just waiting. And it reminds me painfully of, like, that the the dial up modem sound, Like, just waiting.
Andy Hock:And and then you ask yourself, okay. Well, what what happens when things get faster? And you can look at that, dial up versus broadband metaphor. And it turns out that when things get a lot faster, it's not just that the same apps get faster. It's not like we just we we only have billboards or, online, or You're right.
Adam Leventhal:It's not just a thousand x faster BBS or whatever.
Andy Hock:That's right. That's right. Bulletin boards. That's right. It's it's not just thousand x faster than, like, AOL.
Andy Hock:Right? We have we have streaming services. We have social media. There's literally billion dollar industries built on top of sheer performance. So faster doesn't just mean the same stuff goes faster.
Andy Hock:It actually means that people start to build new things. And I think that's what's really cool about this, and and particularly about where Cerebus is in in this space, because we're starting to sort of peek inside that doorway of, like, what would you do if you didn't just have chat, or if your infrastructure didn't just support fast chat, but it also supported things like fast, voice discussions, fast reasoning, fast agentic workflows. All of a sudden, people just start to build some crazy shit on top, that I think is gonna is really gonna open the door to some cool new applications.
Bryan Cantrill:Yeah. That's interesting. Well, it's it's kinda funny because, like, you you on the one hand, like, you you mean, you're absolutely right. You need these kind of these quantum jumps in performance. And I think what's also interesting, because the other thing is that we we didn't hit on, but I think it's important about DeepSeq, is the fact that they were running it on H800s, not H100s, H200.
Bryan Cantrill:And I, you know, I mean, I know there are people who kind of doubt that, but I'm gonna take them it would actually be pretty hard to get a hundred thousand GPUs in country that are that are banned. And the I mean, to me, that that's, like, pretty interesting that they they had to run it with fewer resources. And so, they end up with and the like, just in terms of the the in order to get something that is gonna have a quantum leap of performance, we need to get way more efficient. So we can't actually have this kind of large s of just, you know, the, let's listen to a podcast that referred to this. This is Ed, Ed Zitron.
Bryan Cantrill:Do you listen to him at all?
Adam Leventhal:No. I don't.
Bryan Cantrill:Oh, very funny. Really worth worth listening to him. But, Ed Zitron called they called this the, that OpenAI had, like, the Brewster's Millions, which I'm like, is can you just drop a Brewster's Millions reference? This is, you know okay. I guess that's a the did did that lead to generational chasm?
Bryan Cantrill:The but that OpenAI is kind of this Brewster's Millions problem. The of just, like, we need to spend as much money as we possibly can to justify this kind of and with no focus on efficiency. And the fact that they're getting effectively lapped by someone who had to focus on efficiency because they didn't have a choice, I think it's pretty interesting.
Andy Hock:Yeah. I think this is the era of efficiency. I think this is in both compute and models. I think it's the era of application developers, and it's the era of open source.
Bryan Cantrill:Yeah. Yeah. So, I mean, your view, I mean, certainly, it's our view, but it sounds like your view is, like, open weights definitely here to stay. Yes. There'll be proprietary models to your kind of your Mayo Clinic example, but these general purpose models are gonna increasingly be all open.
Bryan Cantrill:And the I mean, I kinda take them at their word for the motivation, the deep seek motivation of this. I mean, I don't know. Maybe I'm being naive, but I think that, you know, they're like, look. We built this thing on a bunch of open stuff that was out there, and we wanted to give back. Like, makes sense.
Bryan Cantrill:I think it's, the the I I don't know that I completely understand. I mean, it's great, but Facebook's motivation, for being for for having Llama be open. I mean, it is again, it's terrific. I I shouldn't actually you know what? Forget forget acid.
Bryan Cantrill:I actually don't want them to reconsider. Like, wait. What am I doing?
Adam Leventhal:I know. And remember, it's Meta. But yes.
James Wang:I think Meta's strategy is pretty cogent. Right?
Adam Leventhal:Is it?
Bryan Cantrill:Okay. Yeah. Let's oh, yeah. Mhmm. Go ahead.
Bryan Cantrill:Do tell.
James Wang:Like, if you're Zuck and you're investing Do
Bryan Cantrill:I hold on. Hold on. Let me just get my gold chain on here. I'm just so you know, and I need to spend my yacht
James Wang:to get ED over.
Bryan Cantrill:Don't I need to use it? Shirt.
James Wang:If you're Zuck, you wanna, like, commoditize your competitors. Right? And OpenAI has a $20 a month plan, and you have this captive audience called Facebook and Instagram and messenger with 3,000,000,000 people on it. And they have one, they're one click away from a chat box that looks exactly like chat GBT. You can literally give them a free version of that and suck away all the users and then monetize later.
James Wang:That's the classic Facebook playbook since February and whenever,
Adam Leventhal:I guess, and and kind of piss in the pool of everyone trying to to kind of start their new businesses along those lines. No. It makes a ton of sense.
James Wang:Yeah. And and Facebook has enough money, so they don't need to monetize this forever, whereas Sam has to raise every six months. So I think it's actually a pretty brilliant strategy.
Bryan Cantrill:So you you think it is just, purely, and then which, of course, makes sense. I mean, it's like that this is just to make sure that OpenAI can't monetize itself, which is, hey. You know what? It's working. So, I mean, you know, hats off to you.
Bryan Cantrill:I don't know if it's the gold chain or what, but it's, the But
James Wang:I think that you what's amazing, I think, is that that DeepSeek first party has accumulated so much direct consumer demand. That part, like, I would not have guessed in a in a thousand years. Right? Like, topping number one in App Store charts, a Chinese app in the year of Trump, like, none none of those add together. That's not a that's not a coherent story.
James Wang:Like, if that was fiction, you wouldn't believe it. That's how strange it is.
Bryan Cantrill:My my 17 year old, called me. He's like, dad, what do you think about DeepSeek? I'm like, what was everyone asking about DeepSeek now? This is just like and he's like, I, I I even saw that I'm running it right now. And I'm like, you ins you downloaded the model?
Bryan Cantrill:So, yeah, like, it runs really quickly. And I'm like, no. I think you downloaded the app, not the model. Right? It
James Wang:That part is Yeah. He That part is so strange. Like, I didn't think DeepSeek is a reinforcement learning breakthrough. Why would the general populace care about a reinforcement learning breakthrough? I mean, I I don't think the app is even the the end result is not it it barfs a bunch of internal thoughts, but it's not like the end result is dramatically better for everyday queries.
James Wang:Right? Like, if you ask how to make a turkey sandwich, llama's gonna tell you the same thing. I I don't actually know why general people are so interested in it.
Andy Hock:So there's chat g p t moment for a reasoning model?
Bryan Cantrill:But how did it is? What I think is chat g p t I mean, it's, like, $20 a month for people to to for most people have gotta pay for it, or $200 a month, whoever's paying for that.
Andy Hock:But even to you, like, even to this discussion, you're right. It's sort of mysterious because I I think most folks, while they might be AI curious, they don't have any idea what are like, the the the distinction between ChatJ chat g p t and reasoning model. But it's not like they were like, oh, I remember thinking chat g p t was cool a year ago, so now I'm gonna try this deep sea thing because it's a reasoning model. No. I think that's because I live
James Wang:so Exactly. They didn't I guess the question is, what do people think they're getting when they download deep sea? Like, what is the value they're getting?
Andy Hock:They're allowed to participate in the water cooler conversation. I think so. I guess because it because it it just blew up. It came sort of sort of a social media sensation. Right?
Andy Hock:Everybody's asking.
Bryan Cantrill:So I'd
James Wang:be like Yes.
Andy Hock:And, like, if you're I don't know. I'm not a big social media guy. But, like, if you're if you if you need you maybe you feel compelled to write one of those posts that was, like, oh, somebody asked me about deep seek. And so now you have to download it.
James Wang:What's Brian's daughter doing with deep seek? Like, what does she think that it will get from? Yeah.
Bryan Cantrill:That was it was actually my son. My and I would I I do wanna ask him the follow-up question. I mean, he so I would say that, like, the teenagers are definitely all over the large language models, and they use them pretty frequently and not not merely, I mean, but this is the kid that was also, using ChatGPT to write Nextdoor posts to troll Nextdoor, which was, so successful. It was a very short lived experiment because it was just too successful and ultimately it turns out it's very easy to troll Nextdoor and it's very easy. They were having the time of their lives for the for the ninety six hours that before they got booted off.
Bryan Cantrill:So I I don't know. He'd be interested to know kinda where he heard about it, but
Andy Hock:I do think it's like the
Bryan Cantrill:the thing but, you know, especially if it's free, people are gonna be inclined to check it out, I think is the, I so I think
Andy Hock:that's but he did call me.
Bryan Cantrill:He's like, this thing's amazing. I'm like, okay. Well, you should if you you should
Andy Hock:ask it about what it
Bryan Cantrill:thinks about Taiwan and except, he's like, oh, sure. Like, why would it I'm like, well, just ask it. He's like, what's going on? It's telling me that Taiwan's had a catch. I'm like, well, you can You do
Andy Hock:have your fingers on the road
Bryan Cantrill:a little bit on that.
Andy Hock:I really do. That's great. It but I
Bryan Cantrill:I also think it but he but he thought it was good. I mean, he thought it was he's like, I think this is actually better than than ChatGPT. So I don't know. I do think that it, it also I mean, I love the fact that it shows that the the its psychotic reasoning steps just because it reminds me of what Simon had told us earlier, Adam, about the power of running these things on your own is you learn their limitations. And I actually think seeing its chain of thought is helpful for understanding its own limitations.
Bryan Cantrill:And where because I think I'll I mean, I'm still personally trying to figure out, like, how and I feel like in software engineering, we're being, you know, this is a big sea change for us. And I'm still, like, learning about how to make it most useful because it's definitely this idea that you just, like, put it in charge of projects that just goes runs off and does them. That is not my experience.
Adam Leventhal:Totally. Do you find yourself kinda looking retroactively and be like, you know what? This was, like, I wasted time, like, arguing with this thing. Maybe I'm maybe this is oversharing about me. But, like, I've definitely gone through tasks from, like, I should have just buckled down and done it rather than asking those things to do it for me.
Andy Hock:I yeah. Is it because you're not using Cerebras as a back end? That that's it.
Bryan Cantrill:No. Well, that That may be, but I'm
Adam Leventhal:also getting I'm running out of tokens all the time. But I now that I know a guy, I'll see if I can fix it.
Bryan Cantrill:Steven gets more tokens. I know with the streamers again, it's gonna run so fast. I just feel like you're gonna speed run all of software engineering. Be like, no. Like, I I I wrote it.
Bryan Cantrill:It then became a a bloated monolith, and I wasn't able to iterate it at all. So I ripped the whole thing apart, and I've done something that's now that's that's now much smaller, but now this other group is trying to this all happens within, like, you know, hundreds of milliseconds.
Andy Hock:That's right. It publishes and reviews its own articles, and then it follow it was, like, published a follow-up.
Adam Leventhal:Hey. One thing you mentioned earlier was the idea of co design of hardware with these models. And, I I just wanna get back to that and make sure we didn't lose that thread and under because I don't really understand what that means.
Andy Hock:Yeah. I mean, I think, so what I mean when I say co design is, building an algorithm, that, sort of comprehends and takes into account the underlying hardware on which it's run. When you when you think about Yeah. Let's say I'll I'll give you an example from another domain that we that we work in, which is, where where we have users, which is high performance computing that is traditional physics based modeling and simulation. And there, you know, that there are known algorithms to simulate the physics of molecules for things like molecular dynamics, right, for problems like drug design, material design, etcetera, etcetera, etcetera.
Andy Hock:And so this is like a known space. The the physics isn't new, and neither are the algorithms. But, like, running molecular dynamics simulations on GPUs, you only go so fast. We had a a partner in the Department of Energy, do an implementation of the same molecular dynamics physics and algorithm, but designed to optimize our particular chip architecture. Right?
Andy Hock:So effectively, the same calculations, but they took a look at our architecture and said, okay. Well, how can I leverage these compute resources, this communication pattern, or, like, how can I do things differently to optimize the implementation of this computation? Designing the implementation for the underlying hardware. And they they they did that, and they got a really shocking result. They were able to run molecular dynamic simulations on Cerebras, multiple hundreds of times faster than the largest supercomputer on the planet.
Andy Hock:Wow. One one I see. And so I think the the idea is that is say, like, well, look, I'm I'm designing this model, and, and maybe I'll now put it into the sort of hypothetical design space of deep seek. Like, hey, guys. I've, we we wanna develop a state of the art reasoning model.
Andy Hock:We we don't have quite as much data as OpenAI. We don't have a whole lot of state of the art GPUs. How could we do this more efficiently? Right? What what would the model architecture be that could fit on the GPUs that we do have?
Andy Hock:And how could we go about maybe getting access to to data to train the model even though we don't have red light? Let's let's co design the model for the infrastructure that we have. And I think it was in that world where scarcity became the source for innovation.
Bryan Cantrill:Yes. I mean, yeah. Yeah.
Andy Hock:DeepSeek has a smaller, sort of footprint and why they chose a particular mixture of experts architecture so that it could fit and run more efficiently on the GPU infrastructure that they did that they did have. So not only and I don't wanna take away from the the success of that approach. I think it's it's it's remarkable what small teams can do, when they're scrappy and sort of incentivized to be innovative. And in my head, I'm like, I I I wanna get a a team like that working on Cerebras also because I think one of the reasons why we built the Cerebras architecture was to give developers a a better, more purpose built architecture for AI work. And so I'm I'm still waiting for, for folks to build a completely new type of neural network architecture that's optimized for a wafer scale and just take this all to the next level.
James Wang:The closest we've seen to that is the Department of Energy Labs. They're they're the, our best programmers for coding right down to the metal. And every time they get a result, it's it's not in the same digit as what you're using.
Bryan Cantrill:And presumably, that is not and, I mean, that that's not PyTorch. Right? They're they're binding to kind of a low I mean, just in terms of, like, the the Yeah. Getting the the greater power from being at a lower layer of abstraction.
James Wang:It's coding straight to using SDK, which which is which is, yeah, that's a a fewer people, but the, the number, the people who do it get the truly spectacular results. So just the one result, and we published this last year is that the DOE, ran a simulation on a single CS three box, and it was faster than the fastest supercomputer in the world. Yeah. Because it, the fastest computer in the world can't, can literally not make the problem go faster. It can only work on a bigger version of the same problem.
James Wang:Kind of like the difference between strong and weak scaling. But it cannot make a given problem go any faster. Cause if you just distributed eight, like thousands of cores, they're just running the same simulation over and over again, but it can't accelerate that one simulation whereas our machine accelerates the single simulation. It was faster than the fastest computer in the world. That's the kind of result we're looking for.
Bryan Cantrill:Yeah. That's wild. And we and I think it's just to to your your point, Andy, about, like, can as we start thinking about different ways of of now optimizing our models for the things that we actually do have, and just taking a clean sheet of paper, what does that look like? Or clean ish sheet of paper, which it feels like what the DeepSeek folks did to their credit. It's like they actually, like, how do we, and, you know, there's also always an advantage.
Bryan Cantrill:I feel like this is the other kind of, like, a an age old tale that's been retold with DeepSeek that when you are coming to a problem later, you always have an advantage, and you get to not go down some of the blind alleys that earlier folks went down, and you get to quite literally stand on the shoulders of giants. And so you can I mean, the a team of DeepSeek's size, and the resources they had might not have been able to succeed, probably would not have been able to, you know, three years ago? So they, you know, I think we're we're we will, the time is right to kind of take a a clean sheet of paper on a bunch of the stuff.
Andy Hock:Yeah. In fact, I mean, other other cool examples of we know some folks doing, inference optimizations, codesigning for CPU based inference, particularly for Yeah. For small and sparse problems. I think we I I think we probably and we know people that are looking at, new model architectures sort of beyond traditional transformers, like the guys at Liquid AI that are looking at new types of learning models or new types of learning algorithms and model architecture. Sorry.
Andy Hock:But those are I I think, you know, back, you know, a few minutes ago when we talked about, you know, the the the era of efficiency, the era of open source, I think one of the dimensions on which we become more efficient is by the virtue of codesign efforts like this, where we where where teams are looking more closely at the alignment between the neural network architecture and their hardware architecture, and their data and figuring out how to be real scrappy.
Bryan Cantrill:Yeah. Absolutely. Well, I think it's we've got a lot more disruption to come. I think that, speaking personally, I'm definitely, and not that you get Adam and then now that we know a guy that can get us, some more tokens, maybe we can, because it was, it was easy to run out, but it was a lot of fun to go to to play around with. And, well, it feels like other folks can hop on there and play around with with r one, and and you've got a couple of other models on there as well.
Bryan Cantrill:And we see the it is so I gotta tell you, it's so great to see so many things kinda come together. It must be very satisfying for for you both. And, Andy, sounds like you I mean, been there for you've got a a long, many miles of trail behind you, must feel really great to have this really extraordinarily innovative hardware in terms of of a wafer scale and then being able to couple that with these open models, open weight models, and and being able to turn that into something that is, that is this amazing result that it would be a coworker that if Adam discovered their notes, he would go out of the window.
Andy Hock:Run out of the room.
James Wang:No. I would
Andy Hock:I I we we really appreciate the the the invitation, and the card stock. And to your to your point, yeah, this is, you know, it's it's been an incredible run at Cerebras. But I You're not resigning yet. I think I think we have a I'd like to thank everybody. Yeah.
Andy Hock:Like the Academy? I'd like to thank the academy. No. But we we we got in this game, eight, nine years ago, with to to try to build what we thought was the right computing platform for this, you know, what we thought was gonna be a pretty important workload. And I think as as system builders, yeah, there's there's nothing more cool than, and gratifying than seeing people do incredible groundbreaking work on your machine.
Bryan Cantrill:And to have it all actually work. I mean, I don't I don't mean to sound surprised, but, like, it must be the place. It's like No.
Andy Hock:I mean the whole journey,
Bryan Cantrill:but trust me, it didn't always work.
Andy Hock:There there there's a number of ways that this thing could've just caught on
Bryan Cantrill:fire. Tree.
Andy Hock:Exactly. But, but, yeah, just to to to we're we're tool builders, right, at the end of the day. And to to to actually see people using the tool that you've built to to do some incredible stuff, some some work that couldn't have been done with previous tools, There's, there there's nothing more gratifying than that for what we're doing. And so I I but I do think there's just there's a lot more out there. We've only just started.
Andy Hock:And so, yeah, a lot to look forward to, and it's been awesome to be able to to get guys like James aboard that can help us tell the story. And, and we're happy to be here, be able to be a part part of this fun conversation and be able to have something to say about deep seek at the water cooler.
Bryan Cantrill:Awesome. That's great. Well, thank you both. Really, really appreciate it. And, again, congratulations on all the terrific work, and on just the, like, the truly extraordinary performance.
Bryan Cantrill:It's really a lot of fun. And, Adam, thank you for indulging me. I we I felt we really had to do. We we I mean, we had to go in the deep seek. We had to
Adam Leventhal:Absolutely. I mean, I I will say that, like, having had Simon on, you know, a month ago talking about it, I was like, well, what are we gonna talk about again? But there were this was awesome, guys. So so much rich stuff to talk about here, and what you guys have built at Cerebras is remarkable.
James Wang:Really appreciate it. Thanks, Brian, Adam.
Bryan Cantrill:You bet. And then next week, we are European friendly.
Adam Leventhal:I'm sorry. Finally. Right?
Bryan Cantrill:Finally. Yeah. Sorry. But the false alarm for, we we had to had to postpone that from last week, but we're coming up next week. It's gonna be a lot of fun, talking about, Ratatouille, one of our favorite crates.
Bryan Cantrill:So, a a true crate that we love. Awesome. Alright. Thanks, everyone. Talk to you next time.
Creators and Guests
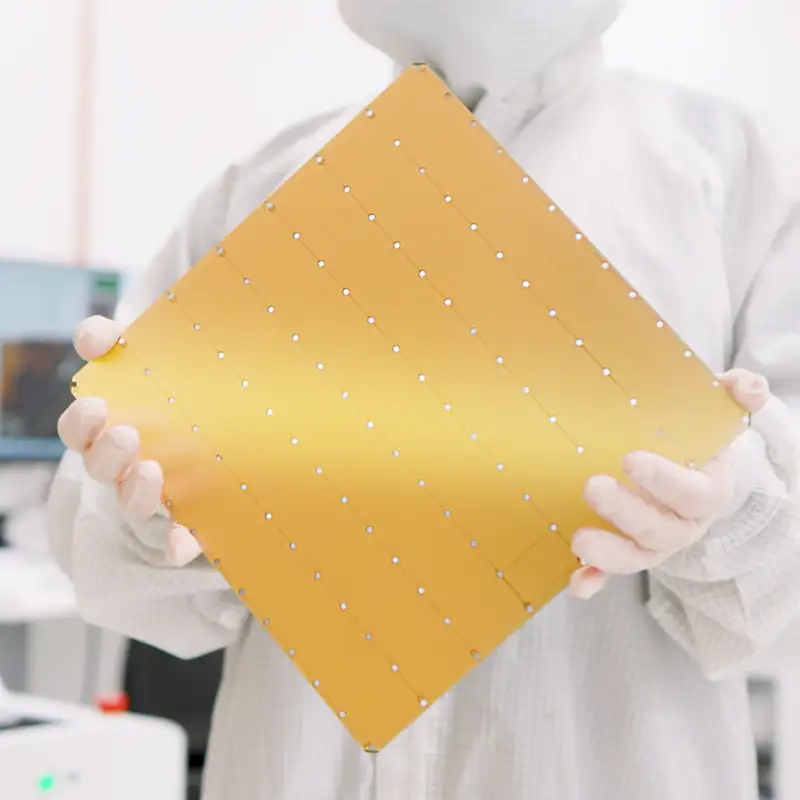